The Humanity of Our AI Future
July 6, 2017
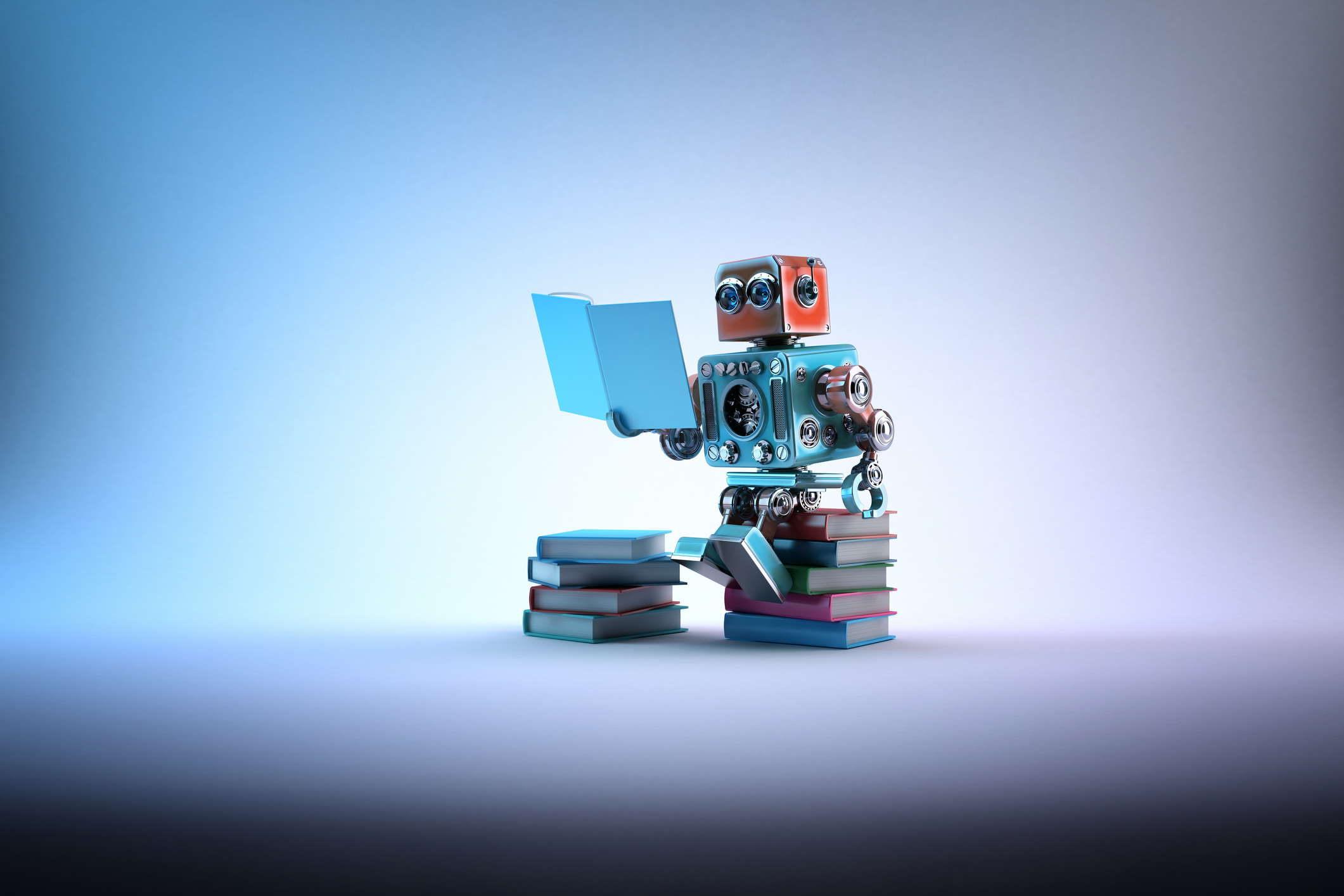
In 1991, as an undergrad, I wrote a paper on social cybernetics and its role in robotics. Out of curiosity, I just did a quick Google search on social cybernetics and found this article comparing and contrasting AI and the study of cybernetics.
In 2017, as a VC, I’m still fascinated by the topic of melding human interactions and feedback mechanisms with the latest advances in technology. I learned early in my career that technology itself doesn’t solve problems – it is the applications of technology that solve problems.
We’ve seen progressions of smarter machines over the years. A new generation, including my niece and nephew who are both under 7 years old, is learning to talk to humans and machines almost interchangeably. We talk to Amazon’s home robot Alexa to ask for the day’s weather, and many of us are more likely to ask Google Home for information than do a Google search on our phone. We’ve been using simplistic robots for years, often without knowing it. Roomba vacuums, launched in 2004, are an example. They incorporated a set of basic sensors that help the devices perform tasks. For instance, the Roomba changed direction on encountering obstacles, and sensed steep drops to keep it from falling down stairs.
Every time we place an order with Amazon.com, robots are responsible for making sure our packages get to us quickly. Robots pick up items on warehouse floors and bring them to human packers. Amazon currently has over 15,000 of these robots across warehouses in the company’s network. What used to take hours of walking can happen in minutes instead. This efficiency is passed on to all of us by lower prices and quicker fulfillment times. E-commerce has grown from $71 billion in sales 10 years ago to $4.5 trillion last year – and machines have enabled much of this growth.
Why have we seen an acceleration of robotic technology over the past 10 years? Both hardware (such as sensors that interact with the environment to collect data) and software (the algorithms that are able to intelligently interact with humans or other machines) have become more sophisticated. Today, we have more data, faster processors and larger computer memories than ever before. As a result, tools such as face recognition, auto-translate and voice-controlled devices have now become more ubiquitous. The computational power available today, in conjunction with the ability to collect and store large amounts of data, allows us to do things that were not possible before.
However, historically, artificial intelligence software has required a lot of training. A human only needs look at a car a few times to recognize the car, but an AI system often needs to process hundreds or thousands of samples before it can retain that information. Machine learning, a subset of AI, creates computer algorithms to automatically learn from data and information – so that computers can adapt and improve their algorithms more autonomously. The next evolution is to turn to deep learning, or cognitive computation, where a machine can learn a task so well that they eventually outperform humans. This will require more processing power (potentially quantum computing) as well as more advanced algorithms.
Given that the human brain is still a black box – with questions still unanswered about consciousness, perception and personality – I believe we are still far away from replacing the human brain. Consider this quote from a NY Times article from 2014:
Dr. Abbott collaborates with scientists at Columbia and elsewhere, trying to build computer models of how the brain might work. Single neurons, he said, are fairly well understood, as are small circuits of neurons.
The question now on his mind, and that of many neuroscientists, is how larger groups, thousands of neurons, work together — whether to produce an action, like reaching for a cup, or to perceive something, like a flower.
As someone who has been interested in the AI space for over 20 years, I am more excited than ever before about how advances in hardware and software, if developed responsibly and with awareness of broader societal impacts of the technology, will enable us to live better lives. As a VC who has built a fund focused on distributed connectivity and data analytics as a thesis, I am realistic about the current status of investment opportunities in the sector. Some of the smartest fledgling teams have been acquired by Google, Apple, Uber and Facebook over the past few years. Entry valuations for these teams were high, often bid up by the interest in the sector (and scarcity of talent). Many teams, while discussing VC investment, were acquired by larger companies for their asking valuations. The lure to sell: working on their technologies, supported by large teams and resources, and unencumbered by constraints of the VC model.
While many of these companies I reference were working on horizontal, platform technologies, I recently have seen more investment opportunities in vertical “applied AI”. Data experts, combined with domain experts, are forming companies to tackle applications that utilize machine learning as core elements of their product. Bradford Cross, a partner at Data Collective, just published a great blog post on this, along with other machine learning predictions for 2017. Many of these teams are focused on sectors such as financial services and healthcare, which already have large data sets to build upon, and which can benefit from the aggregation and analysis of that data to create new services or business models in these sectors. Two private companies that have built impressive scale are Flatiron Health, focused on oncology solutions, which raised $175M led by pharmaceutical company Roche last year; and Kensho, which just announced a $50M round of funding led by S&P Global. I expect many more to come in the next few years.